Exploring PACE’s Role in Cyanobacteria Detection#
Author: Bingqing Liu (University of Louisiana Lafayette)
This tutorial will show you how to use HypterCoast to explore the spectra of a cyanobacteria bloom. We will use blooms in the Gulf of Mexico as an example.
What is HyperCoast?#
HyperCoast streamlines the processing of hyperspectral data from existing spaceborne and airborne missions (e.g., PACE, EMIT, AVIRIS, NEON, and DESIS) and upcoming hyperspectral missions, such as, SBG and GLIMR.
Uncomment the following cell to install the HyperCoast package.
# %pip install hypercoast
# %pip install -U hypercoast
Import libraries
import hypercoast
import pandas as pd
Read PACE level 2 data, which is non-grided nc file.
filepath = "../data/Bingqing/PACE_OCI.20240730T181157.L2.OC_AOP.V2_0.NRT.nc"
dataset = hypercoast.read_pace(filepath)
# Thanks to PACE OCI tutorials and notebooks: https://oceancolor.gsfc.nasa.gov/resources/docs/tutorials/notebooks/oci-file-structure/
plot = hypercoast.view_pace_pixel_locations(filepath, step=20)
dataset
<xarray.Dataset> Size: 2GB Dimensions: (latitude: 1710, longitude: 1272, wavelength: 184) Coordinates: longitude (latitude, longitude) float32 9MB ... latitude (latitude, longitude) float32 9MB ... * wavelength (wavelength) float64 1kB 339.0 341.0 344.0 ... 714.0 717.0 719.0 Data variables: Rrs (latitude, longitude, wavelength) float32 2GB ... Attributes: long_name: Remote sensing reflectance units: sr^-1 standard_name: surface_ratio_of_upwelling_radiance_emerging_from_sea_wat... valid_min: -30000 valid_max: 25000
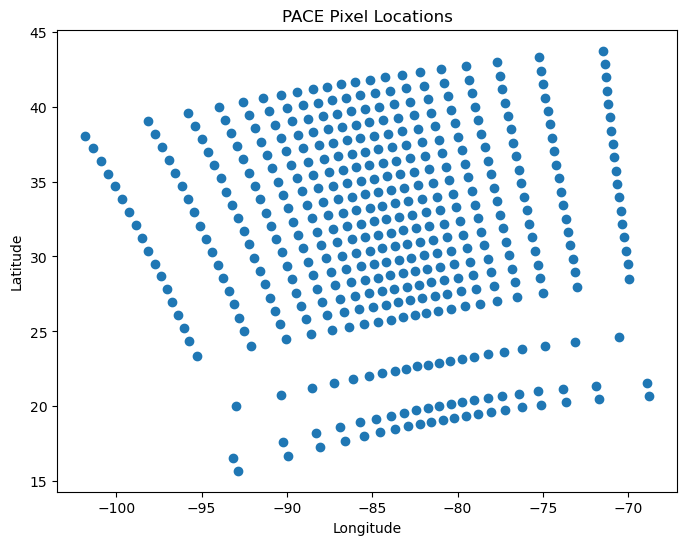
Load field data and interactively display it alongside PACE data.
kml_path = "../data/Bingqing/GOM_Hypoxia.kml"
filepath = "../data/Bingqing/Hypoxia_Data_Sheet.xlsx"
df = pd.read_excel(filepath)
df.head()
Station | Station.1 | Time | Date | Lon | Lat | Depth (m) | Secchi (m) | Salinity | Water Temp | ... | Absorption | CDOM | LISST | Nano | Surface \npH | Sufface \nO2 | Bottom \nO2 | FL-ECO | FL-CDOM | Notes | |
---|---|---|---|---|---|---|---|---|---|---|---|---|---|---|---|---|---|---|---|---|---|
0 | River stations | NaN | NaN | NaT | NaN | NaN | NaN | NaN | NaN | NaN | ... | NaN | NaN | NaN | NaN | NaN | NaN | NaN | NaN | NaN | NaN |
1 | R1 | St1 | 09:39:00 | 2024-07-21 | -89.45114 | 28.89887 | NaN | 2 | 28.71 | 30.74161 | ... | 125ml | yes | yes | no | NaN | NaN | NaN | NaN | NaN | River Mouth |
2 | R2 | St2 | 09:47:00 | 2024-07-21 | -89.45306 | 28.90001 | NaN | 2 | 25.91325 | 30.91475 | ... | 150ml | yes | yes | yes | NaN | NaN | NaN | NaN | NaN | River plume, seaside |
3 | R3 | St3 | 09:59:00 | 2024-07-21 | -89.43833 | 28.89487 | NaN | 0.75 | 24.44862 | 30.59565 | ... | 100ml | yes | yes | yes | NaN | NaN | NaN | NaN | NaN | NaN |
4 | R4 | St4 | 10:13:00 | 2024-07-21 | -89.43162 | 28.90630 | NaN | 0.5 | 8.34838 | 30.13989 | ... | 100ml | yes | yes | yes | NaN | NaN | NaN | NaN | NaN | NaN |
5 rows × 22 columns
df_filtered = df.dropna(subset=['Lon', 'Lat']).reset_index(drop=True)
df_filtered.head()
Station | Station.1 | Time | Date | Lon | Lat | Depth (m) | Secchi (m) | Salinity | Water Temp | ... | Absorption | CDOM | LISST | Nano | Surface \npH | Sufface \nO2 | Bottom \nO2 | FL-ECO | FL-CDOM | Notes | |
---|---|---|---|---|---|---|---|---|---|---|---|---|---|---|---|---|---|---|---|---|---|
0 | R1 | St1 | 09:39:00 | 2024-07-21 | -89.45114 | 28.89887 | NaN | 2 | 28.71 | 30.74161 | ... | 125ml | yes | yes | no | NaN | NaN | NaN | NaN | NaN | River Mouth |
1 | R2 | St2 | 09:47:00 | 2024-07-21 | -89.45306 | 28.90001 | NaN | 2 | 25.91325 | 30.91475 | ... | 150ml | yes | yes | yes | NaN | NaN | NaN | NaN | NaN | River plume, seaside |
2 | R3 | St3 | 09:59:00 | 2024-07-21 | -89.43833 | 28.89487 | NaN | 0.75 | 24.44862 | 30.59565 | ... | 100ml | yes | yes | yes | NaN | NaN | NaN | NaN | NaN | NaN |
3 | R4 | St4 | 10:13:00 | 2024-07-21 | -89.43162 | 28.90630 | NaN | 0.5 | 8.34838 | 30.13989 | ... | 100ml | yes | yes | yes | NaN | NaN | NaN | NaN | NaN | NaN |
4 | R5 | St5 | 10:58:00 | 2024-07-21 | -89.37324 | 28.98095 | NaN | 0.5 | 2.4625 | 30.3054 | ... | 100ml | yes | yes | yes | NaN | NaN | NaN | NaN | NaN | NaN |
5 rows × 22 columns
Visualize PACE image and field data on an interactive map.
m = hypercoast.Map()
m.add_basemap("Hybrid")
wavelengths = [450, 550, 650]
m.add_pace(
dataset, wavelengths, indexes=[3, 2, 1], vmin=0, vmax=0.02, layer_name="PACE"
)
m.add("spectral")
style = {"weight": 2, "color": "red"}
m.add_kml(kml_path, style=style, layer_name="Hypoxia Path", info_mode=None)
m.add_points_from_xy(df_filtered, x="Lon", y="Lat", max_cluster_radius=50, layer_name="Hypoxia Data Points")
m.set_center(-91.46118, 28.89758, zoom=8)
m
Checkout the demo:#
https://i.imgur.com/JdgotIT.gif
Compute band ratios.
da = dataset["Rrs"]
data = (da.sel(wavelength=650) > da.sel(wavelength=620)) & (da.sel(wavelength=701) > da.sel(wavelength=681)) & (da.sel(wavelength=701) > da.sel(wavelength=450))
The spectra of cyanobacteria bloom:#
Visualize the selected region based on band ratios.
import xarray as xr
import matplotlib.pyplot as plt
import cartopy.crs as ccrs
import cartopy.feature as cfeature
# Create a plot
fig, ax = plt.subplots(figsize=(12, 6), subplot_kw={'projection': ccrs.PlateCarree()})
#ax.set_extent([-93, -87, 28, 32], crs=ccrs.PlateCarree())
# Plot the data
data.plot(ax=ax, transform=ccrs.PlateCarree(), cmap='coolwarm', cbar_kwargs={'label': 'Cyano'},)
# Add coastlines
ax.coastlines()
# Add state boundaries
states_provinces = cfeature.NaturalEarthFeature(
category='cultural',
name='admin_1_states_provinces_lines',
scale='50m',
facecolor='none')
ax.add_feature(states_provinces, edgecolor='gray')
# Optionally, add gridlines, labels, etc.
ax.gridlines(draw_labels=True)
plt.show()
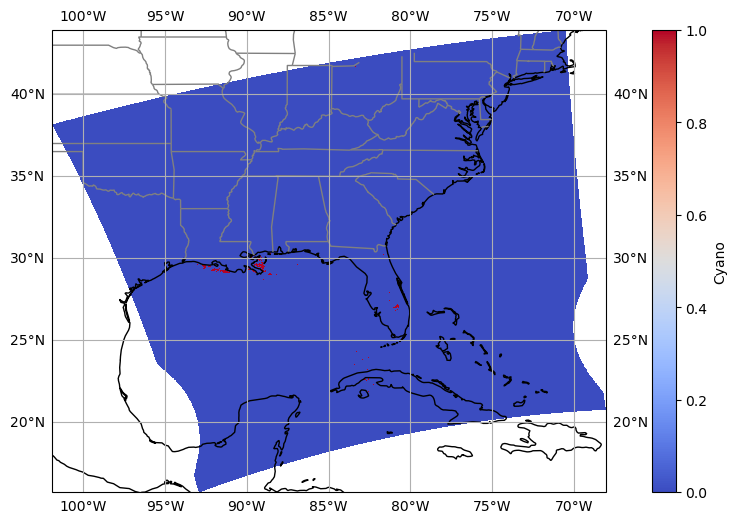
What you can do next about Cyanobacteria using PACE?#
Spectral Angle Mapper: Spectral similarity Input: library of Cyanobacteria bloom Rrs spectra with Chla at different levels
Spectral Mixture Analysis: unmix different cyanobacteria species based on spectral difference.